Inferences
13. Inferences#
In this chapter we return to our roots: Data doesn’t say anything. We say things about data.
The things we say, of course, depend on:
The topics, people, or problems that we decide are worth studying in the first place
How we translate our topic(s) into data, including our overall research design and data collection and measurement strategies
The choices we make in our analyses, including which one(s) to conduct and how to conduct them
The conclusions we deem to be interesting, relevant, or important enough to highlight, share, and later study further
How we make inferences about the real world based on those conclusions
Each of these five steps translates roughly to the shapes in the below highly scientific image – we consider the world, we narrow it down, we conduct an analysis, we draw conclusions, and then we use those conclusion to make inferences about the real world.
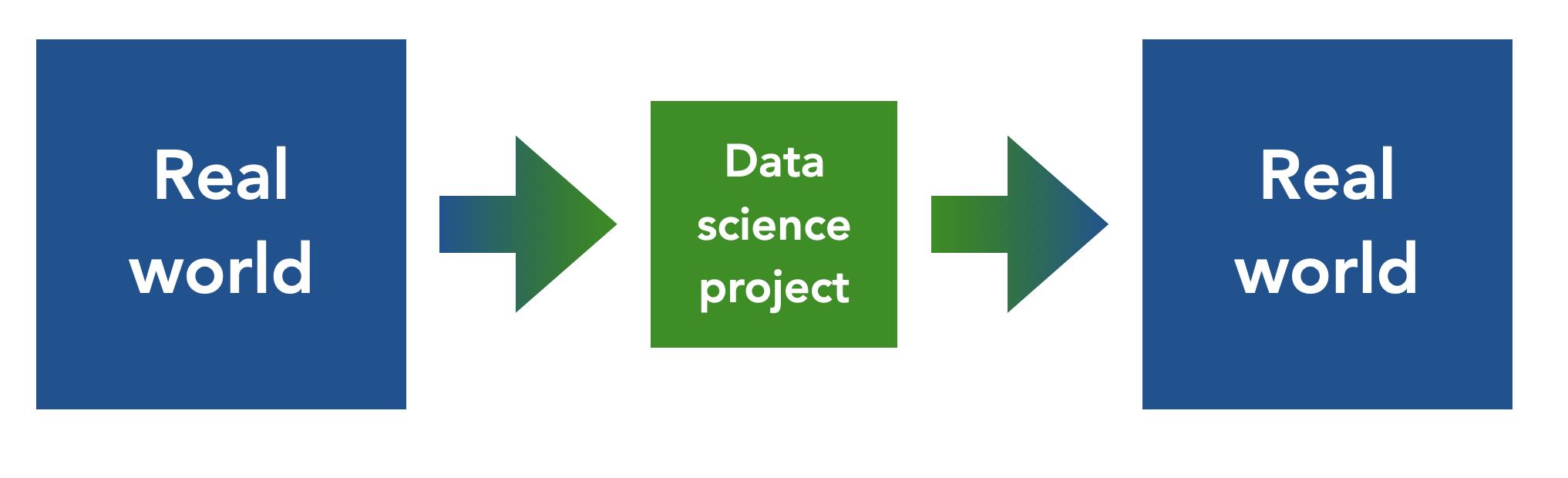
Fig. 13.1 The most nuanced description ever of the data science proceess (images are not to scale)#
Early in our journey in this book – Chapter 2, specifically – we discussed what it means to think like a scientist, and some common areas where we might risk making faulty inferences about the world (even if our work within a study is not technically particularly problematic). You can think of this chapter as something of a bookend to this (also, elegantly, it’s our second-to-last substantive chapter). We will discuss a few other areas where scientists (and everyone) can get tripped up when translating results from a data science project back to the real world (challenges to external validity), even if the project itself is relatively robust (i.e., has internal validity).